Introduction
Hello readers, my name is Daniel and I work as an analytics consultant in the insurance industry. In this post, I wanted to share some of my insights around how data analytics and predictive modeling are revolutionizing the property and casualty insurance business.
Property and casualty insurers have access to massive troves of customer and claims data. However, until recently many insurers were not leveraging this data to its fullest potential. Advances in data science and machine learning are now enabling insurers to gain deeper customer insights and build sophisticated predictive models. These models help insurers better understand risk, improve underwriting accuracy, detect fraud, and enhance the customer experience.
In this post, I will explore how some of the leading P&C insurers are applying cutting-edge analytic techniques across the insurance value chain from marketing and underwriting to claims management. I will also discuss the key challenges and opportunities that data analytics presents for insurers. My aim is to help provide a high-level understanding of this transformational trend to both insurance professionals and general readers interested in learning more.
Let’s dive right in!
Marketing and Customer Acquisition
One of the early areas where insurers started leveraging customer data was in direct marketing and customer acquisition. Proper segmentation allows insurers to target marketing communications and offers to the customers most receptive and likely to purchase. Advanced analytics enable insurers to build highly accurate customer profiles and predictive models of purchase likelihood.
For example, a large auto insurer I consult for built over 200 predictive models just for new customer acquisition alone. Separate models predict the likelihood a prospect will click on an email, visit the website, get an insurance quote, and ultimately purchase a policy. These models take into account thousands of data attributes like demographics, household composition, vehicle type, credit history, existing insurance coverage, marketing channel preferences and more.
The insights from these models help the insurer determine the most cost-effective marketing campaigns and allocate their budgets efficiently. They can invest more on prospects with the highest predicted conversion rates. Complex algorithms also power hyper-personalized messages and content for each prospect. All of this greatly improves response and conversion rates compared to traditional one-size-fits-all approaches.
Underwriting and risk assessment
Once a customer makes contact, underwriting is the next major step in the insurance process. Traditionally underwriters would rely primarily on application information, credit reports and actuarial rate tables to assess risk and determine prices. But insurers are now augmenting human underwriters with advanced algorithms and predictive models.
One major insurer partnered with a data science startup to build over 500 predictive models just for the homeowner insurance line. Models predict everything from claim frequency to severity, premium payment patterns, etc. based on thousands of data points for each policyholder. Attributes include not just application data but education, job history, home value trends, neighborhood crime rates, weather patterns and more.
The models enable hyper-accurate risk scoring at the individual policy level. Underwriters can now identify under-priced risks upfront and price them accordingly or decline risks that are too high. Similarly high-risk customers can be offered additional services or incentives to lower their risk profiles over time. All of this improves underwriting margins and loss ratios.
One agricultural insurer client of mine informed me that their predictive models helped identify $5 million worth of underpriced risks in just the first year. Over three years, their loss ratios dropped 5 points thanks to analytics-driven underwriting. Their actuaries estimate a 10-15% improvement in combined ratios going forward.
Claims Management
On the claims side too insurers are gaining significant benefits from data and analytics. Predictive models now help assess liability and estimate claim severity even before an adjuster physically inspects the loss. Reports indicate models have over 80% accuracy for basic property damage claims.
Fraud detection has seen the biggest success. Predictive algorithms can analyze tens of thousands of attributes for each claim and other “similar” claims to identify anomalous patterns indicating potential fraud. Several insurers confirmed detection rates have doubled or even tripled compared to traditional red-flag approaches.
Identifying fraudulent claims early allows insurers to avoid payouts and significantly brings down expenses. It also ensures legitimate customers receive timely payments. Advanced analytics are also enabling virtual claims adjusting using photos/videos and digital documents instead of onsite visits for smaller claims. This streamlines the process, cuts costs and enhances customer service.
Churn prediction and customer retention
Customer churn, or policy cancellations, pose a major risk for insurers. It costs 5-6 times more to acquire a new customer than retain an existing one. Analytic models built on 2-3 years of customer transaction and interaction data can now predict churn with over 85% accuracy 6 months in advance for many insurers.
One large insurer shared they reduced voluntary annual churn from 18% to 12% by implementing customized retention programs for customers their models identified as high-churn-risk. Strategies included improved service levels, more engaging communications highlighting value, selectively lowering rates and bundling additional products. All of this translated to over $100 million in yearly profit gains.
Predictive strategies are also helping to ‘nudge’ customer behaviors. For example, reminders nudging high-value customers to complete multi-policy or multi-year discounts substantially increased uptake. Detailed usage-based insurance programs incentivized miles reduction for high-risk younger drivers through gamification and rewards. This lowered their overall risk profiles.
Pricing Optimization
With powerful predictive underwriting and risk models in place, insurers can now move towards more dynamic, usage-based and behavior-based pricing approaches. Rather than rely only on broad risk categories, insurers use granular individual-level predictions to set optimal prices.
For auto insurance, pay-per-mile and usage-based programs have taken off. Telematics devices in vehicles capture real-time driving behavior data which influences rates. Safe drivers see discounts as high as 50% compared to traditional policies. Participants also enjoy lower long-term rates by reducing miles or improving their driving habits.
Insurtech startup Metromile generates millions of data points daily from over 150,000 participants. Their models harness this immense stream of driving behavior data to offer hyper-accurate mile-based rates. Early results indicate claim frequencies are 8-15% lower for engaged usage-based insurance customers.
For home insurance, advanced usage-based models are enabling seasonal or temporary coverage discounts. For example, insurers can lower rates for seasonal homes left vacant for part of the year based on property sensors detecting actual occupancy patterns. Rates adjust up automatically when homes are reoccupied, eliminating coverage gaps.
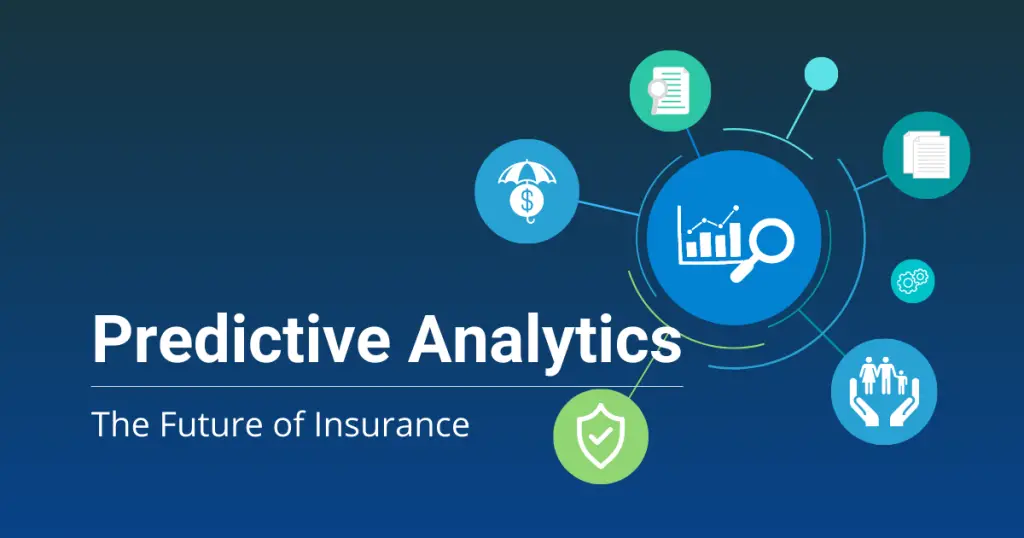
Risk Management and Loss Control
Data-driven risk management focuses on proactively reducing inherent risks to lower future losses instead of just reacting to claims. Predictive models help insurers identify cost-effective risk mitigation strategies.
For example, analysis of past wildfire claims revealed homes built before stricter codes were a major risk factor. Insurers offered discounted rates to these high-risk homeowners contingent on retrofitting with fire-resistant roofs, siding and clearing vegetation. Claimsdropped 50% in subsequent fire seasons in these neighborhoods.
Usage-based driving data also reveals individual risks for accidents like hard-braking, speeding or distracted driving that warrant risk mitigation interventions. Customized risk reduction programs are then delivered through mobile apps and services. Early indications show loss costs decrease 10-15% on average for engaged participants.
Analytics also help pinpoint systemic risks. For example analysis of multiple years of flood claims helped the Federal Flood Insurance program identify and map thousands of previously unreported high-risk areas, allowing better risk management nationwide.
Challenges and Opportunties
While insurers have seen immense value from data and analytics, it is still early days. Significant challenges remain to fully capitalize on its potential. Key issues include:
- Data Integration: Insurance involves data from diverse internal and external sources in disparate formats. Integrating and preparing this “big, messy” data for analytics takes considerable effort and resources.
- Model Interpretability: Explainability of black-box machine learning models is critical for underwriting, audits and compliance in a regulated industry like insurance. Balancing accuracy and transparency remains a challenge.
- Talent Shortage: Building robust analytic capabilities requires data scientists, engineers, research specialists – skills in short supply industry-wide. Retaining talent through effective training, career growth remains critical.
- Vendor Risk: Insurers partnering extensively with Insurtechs and consultants have to diligence vendors adequately to avoid lock-ins, access issues and maintain control over their data assets.
- Privacy & Security: Robust governance frameworks are needed to ensure customer privacy and security as data sharing and usage expands beyond traditional boundaries. Breaches can severely impact trust and reputation.
- Change Management: Large-scale cultural transformation is required to change legacy mindsets and processes and successfully apply analytics at scale across the organization for maximal impact.
- Regulatory Oversight: Evolving regulations around data governance, pricing models and underwriting practices need to keep pace to allow insurers flexibility to innovate responsibly. Complex changes require careful review.
However, there are tremendous untapped opportunities as well. The industry is just scratching the surface of predictive modeling’s full potential. Next frontiers include harnessing massive unstructured data through natural language processing and computer vision. Advances in interpretability will unlock even more value from deep learning models.
Comments
You helped me a lot with this post. I love the subject and I hope you continue to write excellent articles like this.
Your articles are extremely helpful to me. Please provide more information!
Good write-up, I am normal visitor of one抯 site, maintain up the excellent operate, and It is going to be a regular visitor for a long time.
Your comment is awaiting moderation.
Your articles are extremely helpful to me. Please provide more information! http://www.hairstylesvip.com